Ming Li
minglii [AT] umd.edu
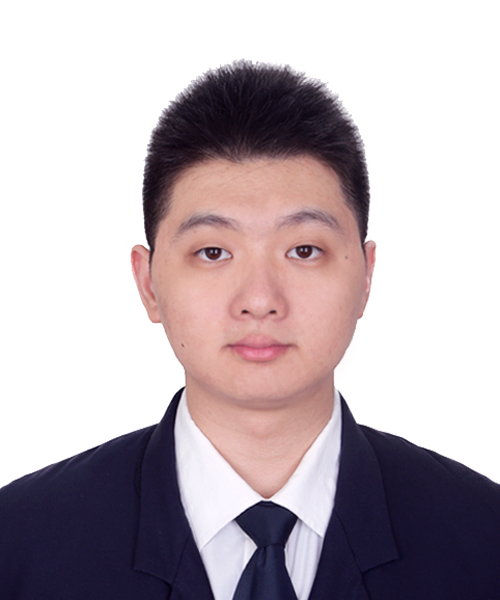
I am a second-year Ph.D. student in Computer Science at the University of Maryland, advised by Prof. Tianyi Zhou. I began my academic journey in computer science with a Bachelor of Science from Xi’an Jiaotong University in 2020, followed by a Master of Science at Texas A&M University advised by Prof. Ruihong Huang in 2023. Besides, I spent 2 years at Shenzhen Institutes of Advanced Technology, Chinese Academy of Science, advised by Prof. Yu Qiao since 2019.
If you are looking for a highly motivated intern with a background in computer science and a passion for advancing AI technologies, I would be thrilled to have an opportunity to chat with you!
My research interests broadly lie in the areas of Machine Learning (ML), Natural Language Processing (NLP), and Large Language Models (LLM).
More specifically, my recent research interests mainly lie in Post-training for LLMs, including:
(i) Data Selection (Cherry LLM (IFD), Superfiltering);
(ii) Data Synthesis (Mosaic-IT, Reflection-Tuning, Selective Reflection-Tuning);
(iii) Controllability (DEBATunE, RuleR);
(iv) Interpretability (Layer_Gradient);
(v) Reasoning (MiP-Overthinking, Gradient_Unified).
I am also interested in and now exploring Vision-LLMs (TRIG, ColorBench), Agent (ATLaS), and RL.
I am really interested in collaboration, feel free to drop me an email for any opportunity!